Why Health Plans Trust the Ribbon Accuracy Model

Why Health Plans Trust the Ribbon Accuracy Model
If we had a nickel for every time we heard “How can I improve my provider data accuracy?” let’s just say… we’d have a lot of nickels. The good news is, you’ve come to the right place for answers. Provider data accuracy is a really hard problem to solve. Research reveals that a staggering 81% of entries across the provider directories of five leading health plans are inaccurate. This isn’t for lack of trying - we know health plans care a lot about improving their provider data as inaccuracies not only affect their members trying to find care but also affect internal business operations, from claims processing to member experience support. This problem is compounded by the increasing regulatory scrutiny on provider data and requirements that change by state and line of business.
That said, there is hope! Over the last 8 years, we’ve worked closely with our health plan customers on a daily basis to achieve significant improvements together, improving member experience, reducing provider abrasion, and considerably reducing operating expenses, all powered by the Ribbon Accuracy Scoring model. What is Accuracy Scoring and why can health plans trust it? Let’s dive in.
Why is maintaining accuracy so hard?
Health plans have a strong stake in ensuring their provider data is correct. This data affects so many downstream use cases across the organization - it impacts the member directory and experience finding care, claims adjudication processes, and network development and contracting - just to name a few. Health plans need to be confident the data is correct so members can quickly and easily find care, and teams can do their jobs without running into errors caused by bad data.
Health plans are clearly invested in ensuring this data is correct - commercial plans alone spend billions of dollars every year trying to update and maintain records. This manual work is a huge operational burden for teams, especially when 30% of provider data churns every year. In reality, health plans are left to manage many rosters from multiple sources, all in varying formats. These rosters often contain errors in basic contact information like the provider’s phone number and misattribute providers to the wrong location, with some rosters suggesting a provider practices at 50+ locations.
On top of that, health plans must comply with various regulations regarding provider directory accuracy, all with different requirements. CMS requires Medicare Advantage plans to contact providers quarterly to verify and update their information, The No Surprises Act requires updates to be made within 2 business days, and each state has its own requirements and schedules for information to be updated. Non-compliance can result in penalties, adding pressure to ensure accuracy.
Regardless of the massive efforts health plan teams are putting in, these organizations are not data companies. An external neutral solution can catch and make sense of all the data noise, and act as a source of record.
How does Ribbon Accuracy Scoring work?
Ribbon Accuracy Scoring is a machine learning model that predicts the accuracy of a given provider record in accordance with CMS-published guidelines - it predicts accuracy across a unique provider NPI, Phone, and Address combination. This results in a quantitative score (from 1-100) that represents the likelihood that a given provider can be found at a location and is contactable using the phone number.
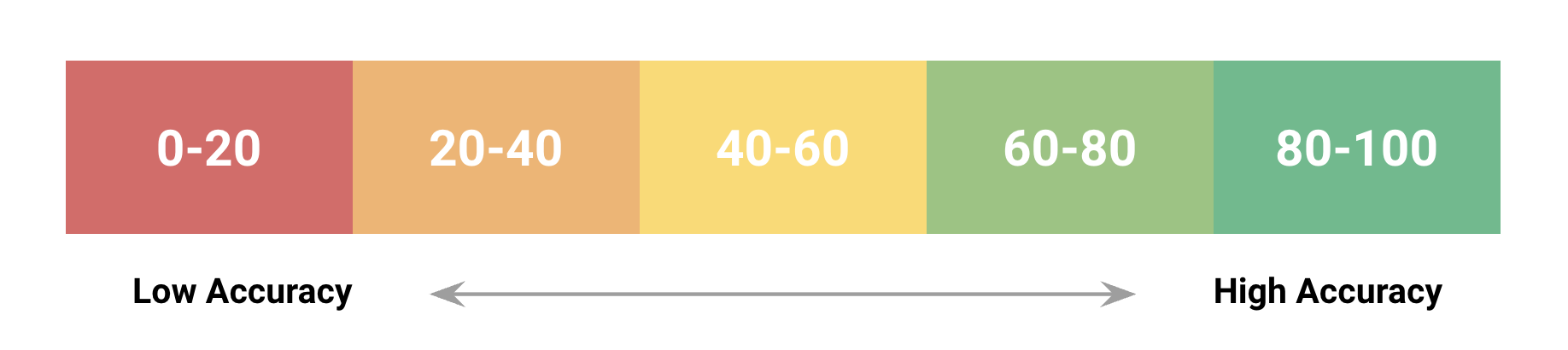
The model is derived from machine learning/AI models that are calibrated from thousands of sources, and factors in several key components, including:
- Data crossover: The number of sources that list the same provider’s address or phone number
- Freshness: The freshness of a data source
- Correctness: The average reliability of the data source
- Completeness: The completeness of the record (i.e. is there a missing phone number?)
The machine learning models that power Ribbon Accuracy Scoring are built on a truth set from provider-validated data collected by our call center. Ribbon continuously performs ongoing back-testing, QA checks, and model refinement to ensure data scoring is accurate. Our testing shows that when we do backtest data through manual validation, our AI-powered Accuracy Scores are correct. As an example, if our model has predicted a record to be 75% accurate, our manual validation shows that the record is in the 70-80% accuracy range.
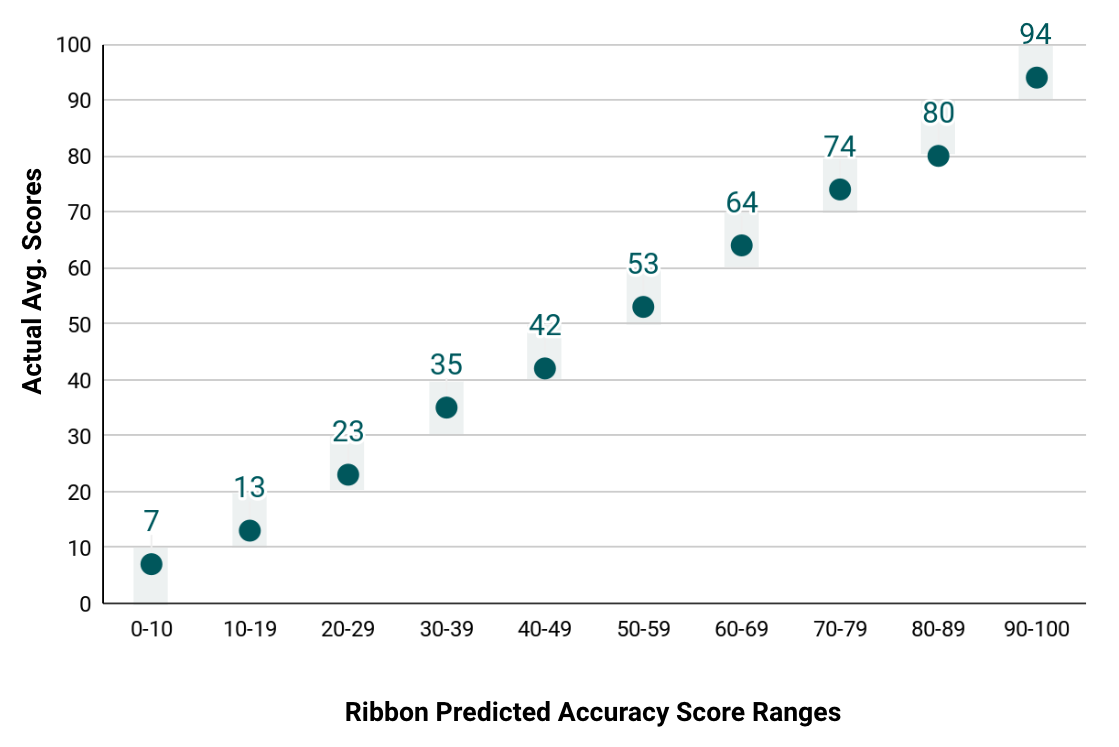
Why do our partners trust Ribbon Accuracy Scores?
Machine learning and AI aren’t buzzwords for our organization - they’re the foundation of how we’ve built our platform and accuracy models. So why do our health plan customers trust Ribbon’s models? There are several reasons our customers trust Ribbon Accuracy Scoring.
- Defining Accuracy: As mentioned above, Ribbon is aligned to the CMS definition of provider data accuracy. CMS’s definition is the industry standard and an important north-star for member experience and meeting compliance requirements.
- Improving Accuracy: Starting during our scoping process, we work with our partners to define an accuracy improvement plan, and then we track it. This looks different for everyone and can require leveraging different components of Ribbon’s platform as challenges and opportunities for improvement vary. Here’s an example of what that process looked like for one Ribbon customer, the Medicare Advantage LOB of a large national payer, in the first 90 days of a partnership.
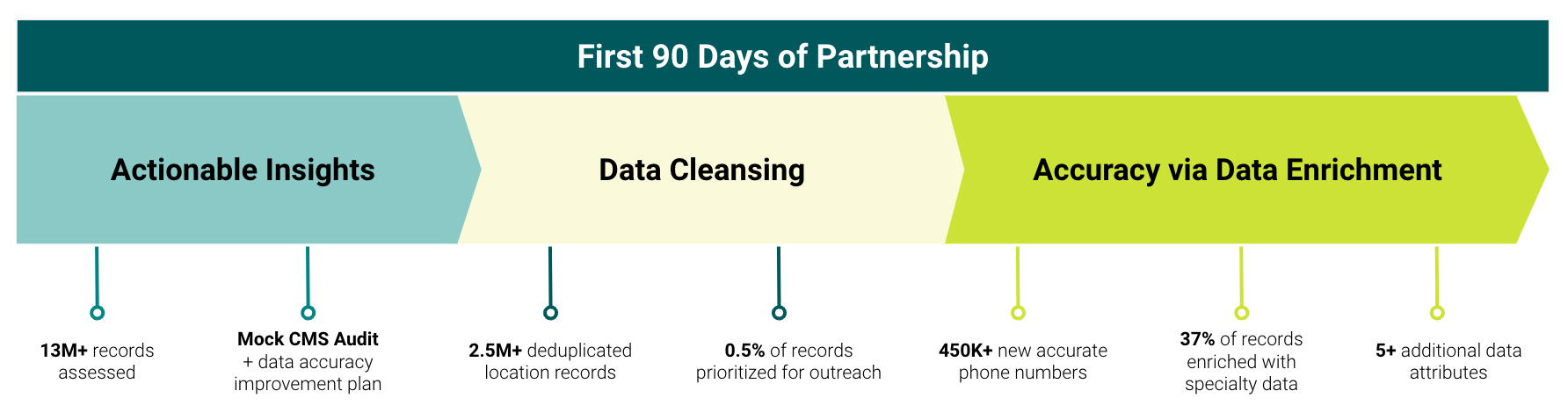
As a result, Ribbon increased the health plan’s directory accuracy by 142% in the first weeks of the partnership.
In the example above, our model classified 80% of the health plan’s records to be low accuracy. One objection we hear from health plans is that if the health plan removes all of these low-accuracy records, they risk not meeting network adequacy requirements. We see this all the time and collaborate with our partners to ensure this isn’t the case. The Ribbon platform can replace those low-accuracy records with high-accuracy records that we have, ensuring the health plan continues to maintain strong coverage. When there are specific network adequacy requirements a health plan is concerned about, we’ll strategically partner to guarantee those are met - for example, manually validate a subset of data rather than automatically suppressing at a certain accuracy threshold.
Here’s an example of the typical steps we take with a health plan to help them hit their accuracy goals.
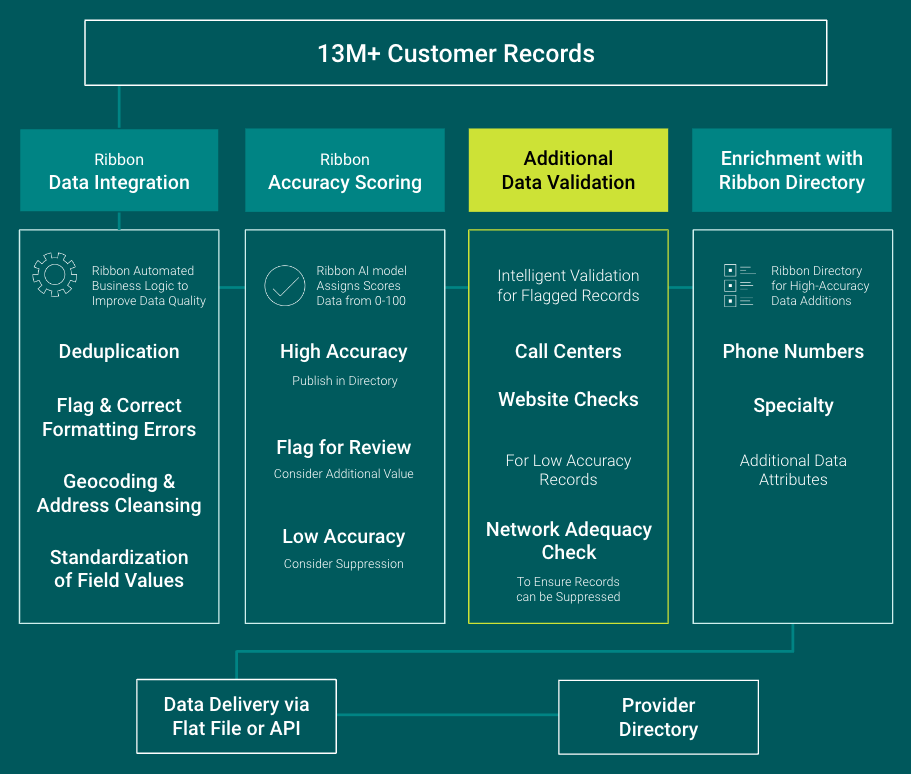
- Evolving the platform: Ribbon is constantly evolving our platform to improve accuracy and ensure transparency. Currently, we’re evolving our data asset to expose which sources are informing which data records - so you’ll know exactly where a phone number, address, etc. is coming from, and the level of accuracy. We are continuously retraining our models so that there’s more data in the two tail ends - i.e. 0-5% accurate and 95-100% accurate, which improves the ability to understand records that are highly likely to be incorrect vs. correct. For example, we recently improved the number of “high-confidence” providers (NPIs with at least one location with a 50%+ Accuracy Score) by 23%. Based on customer needs and priorities, we regularly launch new features and data. We recently launched TINs data within all Ribbon solutions - since many health plans are contracted at the TIN level, this new data allows us to improve the accuracy of the location for a provider, based on locations that are associated with the same TIN a health plan is contracted with.
- Envisioning the future: Our roadmap for the future is robust - beyond just the rest of the year. In addition to traditional AI, we’re exploring generative AI as part of addressing “in-between” records (for example, a 50% Accuracy Score) in an automated way, as opposed to a typical call center. To give you a sneak peek: generative AI (similar to a Chat GPT model) could help with web-researched validation of provider records. This helps to more definitively confirm the accuracy of a provider record and also improves the accuracy of specialties like behavioral health, where updated information is notoriously hard to track down.
Ribbon Expertise
Ribbon has been building our provider data asset and management solutions over the last 8 years, with over 50 customers. Today, two of the top 5 national plans trust Ribbon to improve accuracy and our partners have seen an average of $0.20-0.30 in PMPM savings by implementing Ribbon Provider Data Platform.
Provider data is challenging to get right. It takes the expertise of a team that’s completely dedicated, and data models that have been trained on years and years of data ingestion and processing.
If you’re interested in receiving an assessment on a sample of your data, including recommendations for significantly improving your accuracy, please fill out the form linked below.
Read more about our company and culture
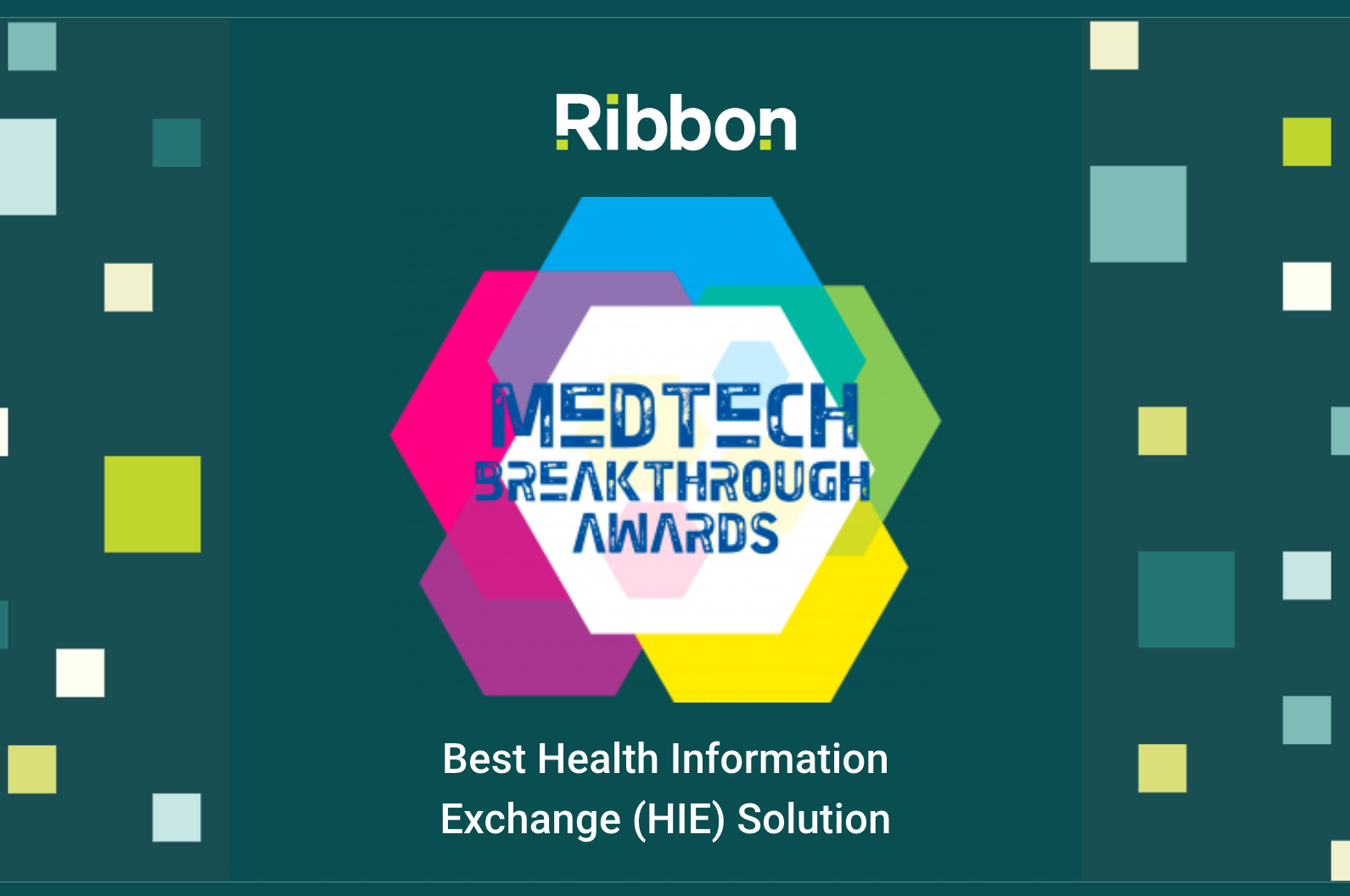